Statistical Machine Learning for Engineering with Applications, Kartoniert / Broschiert
Statistical Machine Learning for Engineering with Applications
Buch
lieferbar innerhalb 2-3 Wochen
(soweit verfügbar beim Lieferanten)
(soweit verfügbar beim Lieferanten)
Aktueller Preis: EUR 85,33
- Herausgeber:
- Anita Schöbel, Jürgen Franke
- Verlag:
- Springer Nature Switzerland, 10/2024
- Einband:
- Kartoniert / Broschiert, Paperback
- Sprache:
- Englisch
- ISBN-13:
- 9783031662522
- Artikelnummer:
- 11998605
- Umfang:
- 400 Seiten
- Nummer der Auflage:
- 2024
- Ausgabe:
- 2024
- Gewicht:
- 604 g
- Maße:
- 235 x 155 mm
- Stärke:
- 22 mm
- Erscheinungstermin:
- 9.10.2024
- Hinweis
-
Achtung: Artikel ist nicht in deutscher Sprache!
Klappentext
This book offers a leisurely introduction to the concepts and methods of machine learning. Readers will learn about classification trees, Bayesian learning, neural networks and deep learning, the design of experiments, and related methods. For ease of reading, technical details are avoided as far as possible, and there is a particular emphasis on applicability, interpretation, reliability and limitations of the data-analytic methods in practice. To cover the common availability and types of data in engineering, training sets consisting of independent as well as time series data are considered. To cope with the scarceness of data in industrial problems, augmentation of training sets by additional artificial data, generated from physical models, as well as the combination of machine learning and expert knowledge of engineers are discussed.The methodological exposition is accompanied by several detailed case studies based on industrial projects covering a broad range of engineering applications from vehicle manufacturing, process engineering and design of materials to optimization of production processes based on image analysis.
The focus is on fundamental ideas, applicability and the pitfalls of machine learning in industry and science, where data are often scarce. Requiring only very basic background in statistics, the book is ideal for self-study or short courses for engineering and science students.
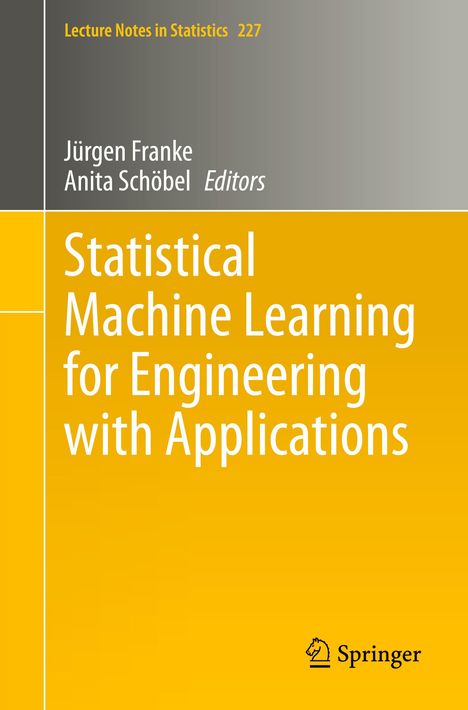
Statistical Machine Learning for Engineering with Applications
Aktueller Preis: EUR 85,33