Maria V. Kulikova: State Estimation for Nonlinear Continuous-Discrete Stochastic Systems, Gebunden
State Estimation for Nonlinear Continuous-Discrete Stochastic Systems
- Numerical Aspects and Implementation Issues
(soweit verfügbar beim Lieferanten)
- Verlag:
- Springer International Publishing, 09/2024
- Einband:
- Gebunden, HC runder Rücken kaschiert
- Sprache:
- Englisch
- ISBN-13:
- 9783031613708
- Artikelnummer:
- 11965317
- Umfang:
- 820 Seiten
- Nummer der Auflage:
- 2024
- Ausgabe:
- 2024
- Gewicht:
- 1379 g
- Maße:
- 241 x 160 mm
- Stärke:
- 50 mm
- Erscheinungstermin:
- 7.9.2024
- Hinweis
-
Achtung: Artikel ist nicht in deutscher Sprache!
Klappentext
This book addresses the problem of accurate state estimation in nonlinear continuous-time stochastic models with additive noise and discrete measurements. Its main focus is on numerical aspects of computation of the expectation and covariance in Kalman-like filters rather than on statistical properties determining a model of the system state. Nevertheless, it provides the sound theoretical background and covers all contemporary state estimation techniques beginning at the celebrated Kalman filter, including its versions extended to nonlinear stochastic models, and till the most advanced universal Gaussian filters with deterministically sampled mean and covariance. In particular, the authors demonstrate that, when applying such filtering procedures to stochastic models with strong nonlinearities, the use of adaptive ordinary differential equation solvers with automatic local and global error control facilities allows the discretization error¿and consequently the state estimation error¿to be reduced considerably. For achieving that, the variable-stepsize methods with automatic error regulation and stepsize selection mechanisms are applied to treating moment differential equations arisen. The implemented discretization error reduction makes the self-adaptive nonlinear Gaussian filtering algorithms more suitable for application and leads to the novel notion of accurate state estimation.
The book also discusses accurate state estimation in mathematical models with sparse measurements. Of special interest in this regard, it provides a means for treating stiff stochastic systems, which often encountered in applied science and engineering, being exemplified by the Van der Pol oscillator in electrical engineering and the Oregonator model of chemical kinetics. Square-root implementations of all Kalman-like filters considered and explored in this book for state estimation in Ill-conditioned continuous¿discrete stochastic systems attract the authors¿ particular attention.
This book covers both theoretical and applied aspects of numerical integration methods, including the concepts of approximation, convergence, stiffness as well as of local and global errors, suitably for applied scientists and engineers. Such methods serve as a basis for the development of accurate continuous¿discrete extended, unscented, cubature and many other Kalman filtering algorithms, including the universal Gaussian methods with deterministically sampled expectation and covariance as well as their mixed-type versions. The state estimation procedures in this book are presented in the fashion of complete pseudo-codes, which are ready for implementation and use in MATLAB® or in any other computation platform. These are examined numerically and shown to outperform traditional variants of the Kalman-like filters in practical prediction / filtering tasks, including state estimations of stiff and / or ill-conditioned continuous¿discrete nonlinear stochastic systems.
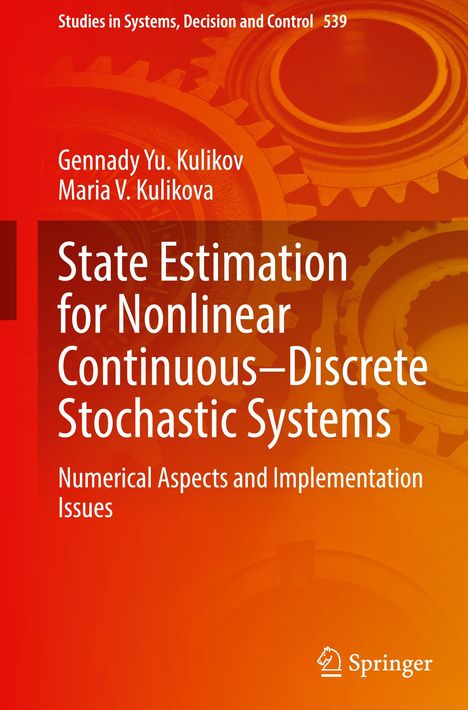