Machine Learning for Causal Inference
Machine Learning for Causal Inference
Buch
- Herausgeber: Zhixuan Chu, Sheng Li
- Springer International Publishing, 11/2023
- Einband: Gebunden, HC runder Rücken kaschiert
- Sprache: Englisch
- ISBN-13: 9783031350504
- Bestellnummer: 11698248
- Umfang: 316 Seiten
- Nummer der Auflage: 23001
- Auflage: 1st ed. 2023
- Gewicht: 641 g
- Maße: 241 x 160 mm
- Stärke: 23 mm
- Erscheinungstermin: 26.11.2023
Achtung: Artikel ist nicht in deutscher Sprache!
Klappentext
This book provides a deep understanding of the relationship between machine learning and causal inference. It covers a broad range of topics, starting with the preliminary foundations of causal inference, which include basic definitions, illustrative examples, and assumptions. It then delves into the different types of classical causal inference methods, such as matching, weighting, tree-based models, and more. Additionally, the book explores how machine learning can be used for causal effect estimation based on representation learning and graph learning. The contribution of causal inference in creating trustworthy machine learning systems to accomplish diversity, non-discrimination and fairness, transparency and explainability, generalization and robustness, and more is also discussed. The book also provides practical applications of causal inference in various domains such as natural language processing, recommender systems, computer vision, time series forecasting, and continual learning. Each chapter of the book is written by leading researchers in their respective fields.Machine Learning for Causal Inference explores the challenges associated with the relationship between machine learning and causal inference, such as biased estimates of causal effects, untrustworthy models, and complicated applications in other artificial intelligence domains. However, it also presents potential solutions to these issues. The book is a valuable resource for researchers, teachers, practitioners, and students interested in these fields. It provides insights into how combining machine learning and causal inference can improve the system's capability to accomplish causal artificial intelligence based on data. The book showcases promising research directions and emphasizes the importance of understanding the causal relationship to construct different machine-learning models from data.
Biografie (Sheng Li)
Sheng Li wurde 1975 in Taiyuan, in der VR China geboren, wo er sein Studium im Fach Jura absolvierte und schließlich zwei Jahre lang als Dozent für Wirtschaftsrecht an der juristischen Fakultät der North University of China arbeitete Zurzeit arbeitet er an seiner Promotion an der Universität Bayreuth.§Als chinesischer Rechtswissenschaftler in Deutschland sieht er es als seine Aufgabe, einen Beitrag zum Austausch zwischen deutschem und chinesischem Recht zu leisten. Von März bis Juli 2010 arbeitete er als Gutachter im Programm Verbraucherschutz und Produktsicherheit bei der GIZ China und wirkte beim Deutsch-Chinesischen Symposium zur Spielwarensicherheit mit. Die Tätigkeit motivierte ihn, sich der Thematik des vorliegenden Buches zu widmen.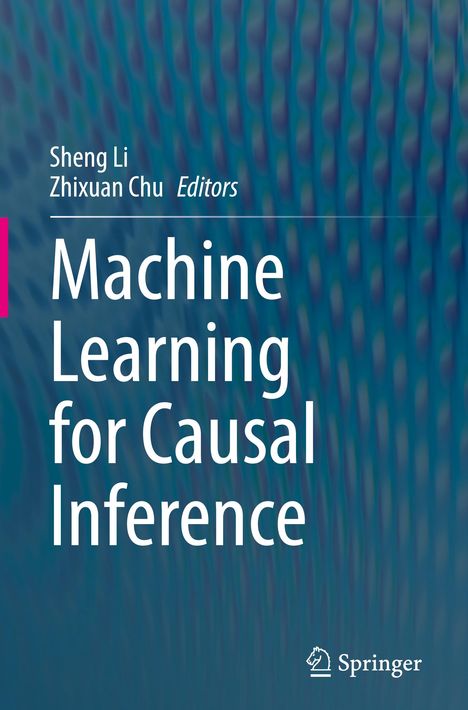
Machine Learning for Causal Inference
EUR 164,28*