Antonio Galves: Probabilistic Spiking Neuronal Nets, Kartoniert / Broschiert
Probabilistic Spiking Neuronal Nets
- Neuromathematics for the Computer Era
(soweit verfügbar beim Lieferanten)
- Verlag:
- Springer International Publishing, 10/2024
- Einband:
- Kartoniert / Broschiert, Paperback
- Sprache:
- Englisch
- ISBN-13:
- 9783031684081
- Artikelnummer:
- 12030835
- Umfang:
- 216 Seiten
- Gewicht:
- 335 g
- Maße:
- 235 x 155 mm
- Stärke:
- 12 mm
- Erscheinungstermin:
- 17.10.2024
- Hinweis
-
Achtung: Artikel ist nicht in deutscher Sprache!
Klappentext
This book provides a self-contained introduction to a new class of stochastic models for systems of spiking neurons. These systems have a large number of interacting components, each one evolving as a stochastic process with a memory of variable length. Several mathematical tools are put to use, such as Markov chains, stochastic chains having memory of variable length, point processes having stochastic intensity, Hawkes processes, random graphs, mean field limits, perfect sampling algorithms, the Context algorithm, and statistical model selection. The book¿s focus on mathematically tractable objects distinguishes it from other texts on theoretical neuroscience. The biological complexity of neurons is not ignored, but reduced to some of its main features, such as the intrinsic randomness of neuronal dynamics. This reduction in complexity aims at explaining and reproducing statistical regularities and collective phenomena that are observed in experimental data, an approach that leads to mathematically rigorous results.
With an emphasis on a constructive and algorithmic point of view, this book is directed towards mathematicians interested in learning about stochastic network models and their neurobiological underpinning, and neuroscientists interested in learning how to build and prove results with mathematical models that relate to actual experimental settings.
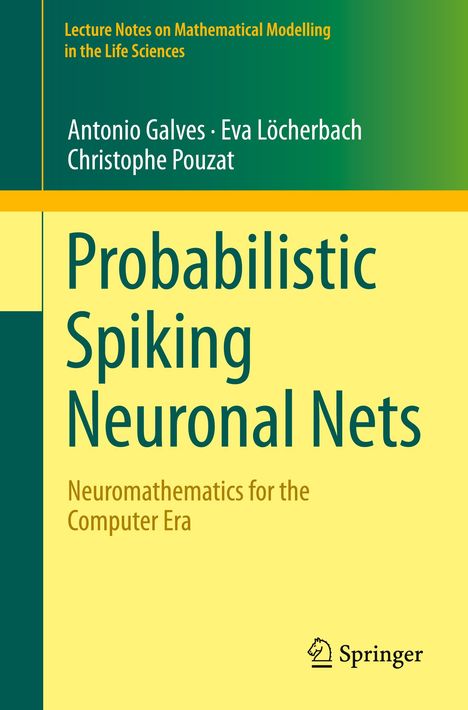